在 单次遍历,等概率随机选取问题 中已经剧透了今天的内容,那就是带权随机选取(Weighted Random Sample)问题。
问题描述:有一组数量未知的数据,每个元素有非负权重。要求只遍历一次,随机选取其中的一个元素,任何一个元素被选到的概率与其权重成正比。
设元素总数为 n,当然在遍历结束前 n 是未知的。设第 i(1 <= i <= n)个元素的权重为 wi(> 0),则权重总和为 \(w=\sum_{i=1}^{n}{w_i}\),也是在遍历结束时才知道的。根据题目要求,第 i 个元素被选取的概率应该等于 \(p_i=\frac{w_i}{w}\)。
虽然加了个权重,但解法依旧非常简单,在 单次遍历,等概率随机选取问题 中的 RandomSelect 函数上稍作修改就得到本问题的解法,依旧是 O(n) 时间,O(1) 辅助空间:
1 2 3 4 5 6 7 8 9 10 11 12 13 | from random import Random
def WeightedRandomSelect(rand=None):
selection = None
totalweight = 0.0
if rand is None:
rand = Random()
while True:
# Outputs the current selection and gets next item
(item, weight) = yield selection
totalweight += weight
if rand.random() * totalweight < weight:
selection = item
|
其中 Python 的 random.random() 返回 [0, 1) 之间的随机小数。
看一下是否满足概率要求。如果最终被选取的是第 i(1 <= i <= n)个元素,那必须是遍历到它的时候,恰好被选中,即 rand.random() * totalweight < weight,其概率为:
另外,还要在处理以后的任何一个元素时,第 i 个元素都没有被替换掉,即对于任意的 j(i < j <= n),第 j 个元素都不会被选中,其概率为:
因此,第 i 个元素最终被选取的概率为:
下面这段程序调用 WeightedRandomSelect 对一组具有等差数列权重的元素进行选取。
1 2 3 4 5 6 7 8 9 10 11 12 13 14 15 16 | # Sample code to use WeightedRandomSelect function
# Use an arithmetic sequence as weights
n = 10
# weights are [1, 2, 3, ..., 10]
weights = [i + 1 for i in xrange(n)]
repeat = 100000
occurrences = [0 for i in xrange(n)]
rand = Random()
for i in xrange(repeat):
selector = WeightedRandomSelect(rand)
selector.next()
selection = None
for item in xrange(n):
selection = selector.send((item, weights[item]))
occurrences[selection] += 1
print occurrences
|
某次运行结果为:
[1723, 3644, 5405, 7326, 9027, 10903, 12678, 14784, 16345, 18165]
而对于这组权重的概率理论值为:
1 : 2 : 3 : 4 : 5 : 6 : 7 : 8 : 9 : 10
= 0.0181818 : 0.0363636 : 0.0545455 : 0.0727273 : 0.0909091 : 0.109091 : 0.127273 : 0.145455 : 0.163636 : 0.181818
可见程序是正确的。
扩展:选取 m 个元素,概率理论值
来看看选取多个元素的问题。当选取多个元素时,可以认为选取过程是逐步进行的,即无放回的多次选取。每一次选取时,任何一个元素被选中的概率都与其权重成正比,但总的权重则又剩余的元素集合决定。
当 m=2 的时候,第 i 个元素被选中可以是两种情况:第一次就被选中;第一次未被选中,第二次被选中。可以得到其概率为这两种情况的概率之和,即:
值得注意的是,即便 wi 和 w 不变,如果其他元素的概率分布不同,最后得到的结果也不同,因此上面这个式子无法把其中的求和化简掉。
从另一方面来看,第 i 个元素被选中的概率等于 1 减去它不被选中的概率。用 \(\bar p\) 表示不被选中的概率,则有:
显然,\(p_i(2)+\bar p_i(2)=1\)。
当 m>2 时,其概率表达式将会变得异常复杂,因为跟概率分布有关,所以算式无法化简。未被选中的概率计算式要稍微简单些,大概是这个样子的:
其中,\(\forall 1\leq k\leq m,j_k\notin\{i,j_1,j_2,\cdots,j_{k-1}\}\)。
对于给定的一组权重,可以用下面这段程序计算出任意 m、i(程序中的 i 是从 0 开始的)对应的概率数值(请无视其 coding style):
1 2 3 4 5 6 7 8 9 10 11 12 13 14 15 16 17 18 | def Foo(weights, ids, totalweight, m, i, times):
if times == m: return 1
p = 0.0
for j in ids:
ids.remove(j)
p += float(weights[j]) / totalweight \
* Foo(weights, ids, totalweight - weights[j], m, i, times + 1)
ids.add(j)
return p
def CalcSampleProbability(weights, m, i):
n = len(weights)
assert 0 <= i < n, 'invalid i'
assert 0 < m <= n, 'invalid m'
ids = set(xrange(n))
ids.remove(i)
p = Foo(weights, ids, sum(weights), m, i, 0)
return 1 - p
|
可惜算法的复杂度非常高,CalcSampleProbability 需要 O(n^m) 时间来完成一次计算。期待高手改进。
来看一下等权重、等差数列权重和等比数列权重的 n 选 m 概率分布图(图中 i 依旧采用 1 <= i <= n 的取值范围):
Mathematica 提供了 RandomSample 函数,支持带权选取,当然它是在遍历之前就已经知道元素个数的。给它一组等差分布的权重,可以看出十万次随机选取后得到的概率分布与上面的理论分布非常接近。
count = 10;
weights = Range[count];
elems = Range[count];
retry = 100000;
map = Table[
freq = ConstantArray[0, count];
For [i = 0, i < retry, i++,
freq += BinCounts[RandomSample[weights -> elems, m], {1, count + 1, 1}]
];
freq, {m, 1, count, 1}
];
ListLinePlot[map / retry, PlotMarkers -> Automatic]
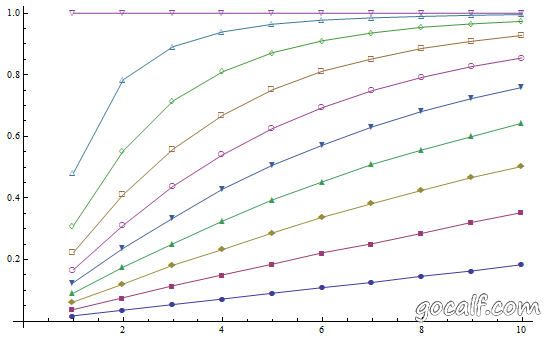
Mathematica RandomSample 随机选取 m 个元素,第 i 个元素被选中的概率
苦苦思考了好几天,但并没有想到一个直观的方法可以给之前的 RandomSample 加上权重处理。因为那概率式子太复杂,实在不知道该怎么去凑。不过在下一篇文章中将会介绍一个神奇的算法(当然不是我想出来的),并且会给出我的证明。
现在发文章的速度越来越慢了,实在因为能力有限,每次为了一两个式子都要演算很久。再接再厉。
Comments
So what do you think? Did I miss something? Is any part unclear? Leave your comments below.